Enhancing Cybersecurity with Machine Learning Malware Detection
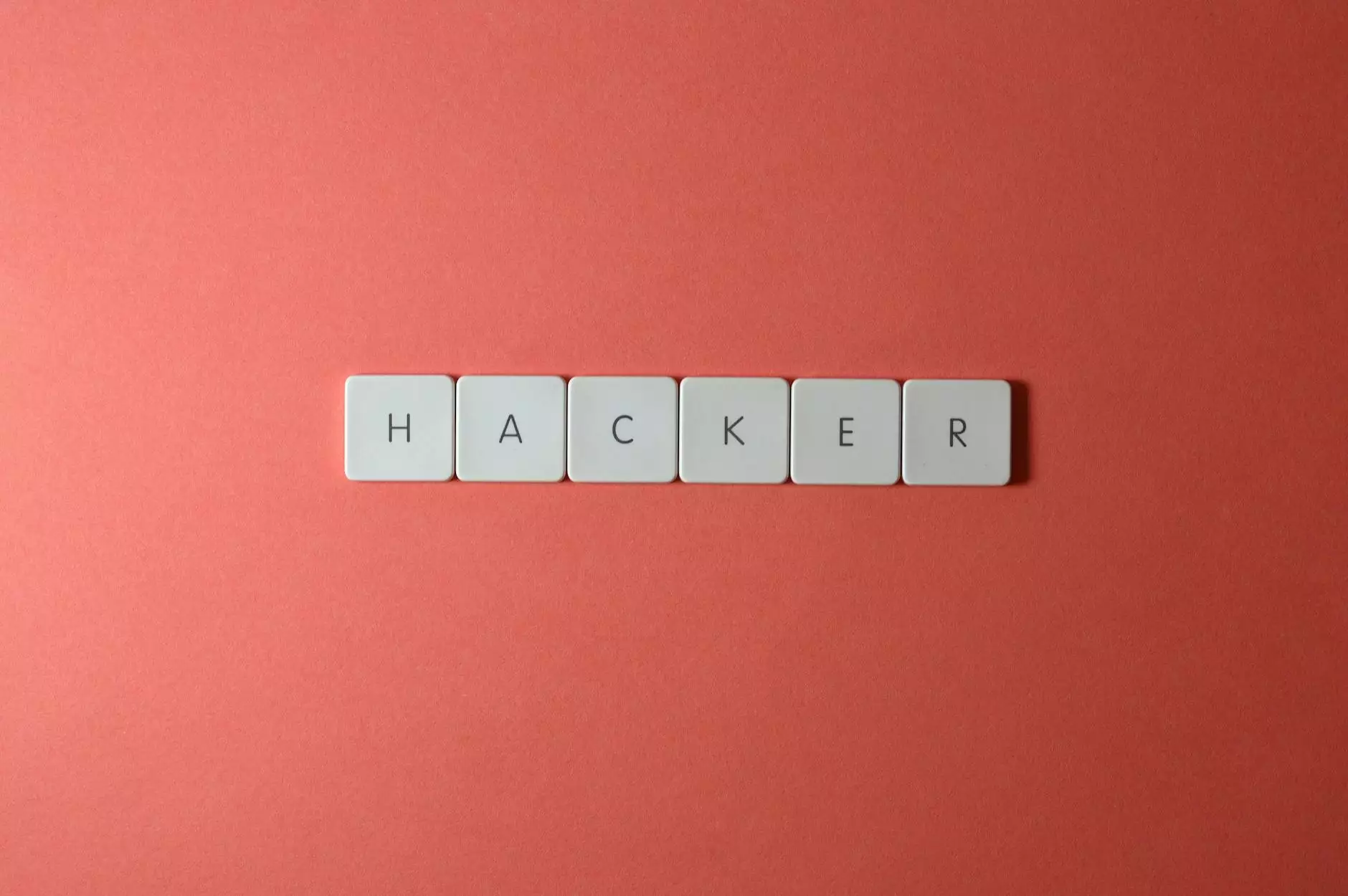
In today's digital landscape, where the threat of cyberattacks looms large, businesses must adopt proactive measures to safeguard their sensitive information and operational integrity. One of the most revolutionary advancements in this field is the advent of machine learning malware detection. This cutting-edge technology empowers organizations to identify and neutralize threats more efficiently than ever before.
The Rise of Cyber Threats
Cyber threats continue to evolve at a staggering pace, with attackers employing increasingly sophisticated strategies. According to recent statistics, cybercrime is projected to cost businesses around $10.5 trillion annually by 2025. These alarming figures underline the necessity for organizations to prioritize their cybersecurity strategies. They need to adapt to new threats by leveraging advanced technologies like machine learning.
Understanding Malware
Malware, short for malicious software, encompasses any software intentionally designed to cause harm to a computer, server, client, or network. This includes various types of threats such as:
- Viruses: Malicious programs that replicate and spread from one computer to another.
- Worms: Self-replicating malware that spreads across networks without human action.
- Trojans: Malicious software disguised as legitimate software.
- Ransomware: Malware that locks users out of their files and demands payment for restoration.
What is Machine Learning?
Machine learning is a subset of artificial intelligence that allows systems to learn from data and improve their performance over time without being explicitly programmed. In the context of cybersecurity, machine learning analyzes vast amounts of data to detect patterns and anomalies associated with malware behavior.
How Does Machine Learning Malware Detection Work?
Machine learning malware detection incorporates several key processes:
- Data Collection: Gathering data from various sources, including system logs, network traffic, and user behaviors.
- Feature Extraction: Identifying relevant features from the data that may indicate malicious activity.
- Model Training: Using historical data to train machine learning models to recognize patterns specific to malware.
- Detection and Response: Deploying the trained models to monitor real-time data for suspicious activities and responding accordingly.
The Advantages of Machine Learning Malware Detection
The integration of machine learning into malware detection brings several significant benefits:
1. Proactive Threat Identification
Machine learning enables organizations to identify threats before they cause harm. By continually analyzing data, these systems can spot suspicious behavior in real-time, allowing for quick action.
2. Adaptive Learning
One of the standout features of machine learning systems is their ability to adapt. As new malware variants emerge, these systems learn from new data and update their detection protocols, which traditional methods may not be equipped to handle.
3. Reduced False Positives
Machine learning algorithms can significantly reduce the number of false positives compared to traditional signature-based detection methods. By considering contextual data, these systems can differentiate between benign and malicious activities more accurately.
4. Scalability
As a business grows, so does its data. Machine learning solutions can scale effortlessly to handle larger datasets, making them ideal for organizations of any size.
5. Efficiency
Automating the malware detection process through machine learning frees up IT resources. Cybersecurity teams can focus on strategic initiatives rather than manually analyzing and responding to every potential threat.
Implementing Machine Learning Malware Detection in Your Organization
Organizations keen on enhancing their cybersecurity posture should consider the following steps to implement machine learning malware detection effectively:
1. Assess Current Security Infrastructure
Evaluate your existing security systems to identify gaps and understand where machine learning can provide the most value. Ensure that your systems can integrate well with new technologies.
2. Choose the Right Machine Learning Solutions
There are numerous machine learning solutions available tailored for various needs. Look for reputable vendors or consider developing custom solutions that align with your specific requirements. Ensure they have robust malware detection capabilities.
3. Data Preparation
Data is the backbone of machine learning. Collect, clean, and organize data from relevant sources to train your models effectively. The quality of data significantly influences the performance of the algorithms.
4. Continuous Monitoring and Improvement
Post-implementation, continuously monitor the system’s performance. Utilize feedback to improve the models and adapt to evolving threats, keeping your system relevant and effective.
Case Studies: Successful Implementations of Machine Learning Malware Detection
Various organizations have successfully deployed machine learning malware detection, resulting in robust defenses. Here are a couple of noteworthy examples:
1. Company A - Financial Sector
Company A, a leading financial institution, implemented a machine learning solution that reduced malware incidents by 70%. By accurately identifying and blocking threats in real-time, the company saved millions in potential damages.
2. Company B - E-Commerce
Company B, an e-commerce giant, utilized machine learning to combat increasing credit card fraud. Their implementation led to an 85% reduction in fraud cases by employing anomaly detection techniques, significantly enhancing customer trust.
The Future of Machine Learning in Cybersecurity
The rapid pace of technological advancement suggests that machine learning will play an increasingly critical role in cybersecurity. As cyber threats become more sophisticated, continuous investment in machine learning solutions will be vital for protecting businesses against attacks.
Conclusion
Machine learning malware detection is not just a trend but a vital component of modern cybersecurity strategies. By adopting this technology, businesses can significantly enhance their defenses against a diverse range of cyber threats. Investing in machine learning solutions becomes not only a smart decision but a necessary step towards ensuring the resilience and security of your organization.
For comprehensive IT services and computer repair, coupled with state-of-the-art security systems, consider partnering with Spambrella.com. Our solutions are designed to keep your enterprise secure, leveraging the finest technologies available in today’s market.